Introduction: The Data Dilemma
In 2018, researchers working on training one of the first widely-used large language models at OpenAI realized something unsettling: they were running out of the internet.
Okay—maybe not literally out of the internet. But they had scraped every available public Reddit thread, Wikipedia article, news site, forum post, and digitized book they could legally get their hands on. Their massive model was still hungry, and the remaining data was either low-quality, copyrighted, or riddled with ethical and privacy concerns.
Fast forward to today, and the problem has only grown more urgent. With the explosion of generative AI, more companies than ever are racing to build their own models—chatbots, assistants, copilots, tutors, therapists, even dungeon masters. The catch? They’re all drinking from the same data pool… and that pool is drying up.
A 2022 study by researchers at Epoch projected that we’ll hit a hard cap on high-quality, publicly available training data as early as 2026 (Villalobos et al., 2022). That means within the next year or two, many AI companies will face a major bottleneck—not from compute limits, not from lack of funding—but from an absence of words to train their machines with. Talk about an ironic twist for language models.
As AI companies dig deeper into every corner of the web, they encounter another problem: most of the internet isn’t that great. It’s noisy, repetitive, biased, and full of misinformation. And more importantly, it’s human. Which means it’s limited. Legally, ethically, and creatively.
This has led to one of the most interesting—and slightly sci-fi—turns in the story of AI: using artificial intelligence to generate more of the very data it needs to learn. A sort of bootstrapping intelligence. An infinite mirror of knowledge. In short: synthetic data.
What is Synthetic Data?
This has led to one of the most interesting—and slightly sci-fi—turns in the story of AI: using artificial intelligence to generate more of the very data it needs to learn. A sort of bootstrapping intelligence. An infinite mirror of knowledge. In short: synthetic data.
But what is synthetic data, really?
Think of it like this: instead of collecting real photos of cats and dogs to teach a model how to tell them apart, you use a powerful AI to create realistic-looking (but entirely fake) images of cats and dogs. Then you use those to train your model. No photo shoots. No permission slips. No actual fur involved.
Synthetic data is artificially generated data that mimics real-world information. It can take the form of text, images, audio, video, code—anything that a model might need to learn from. The key is that it’s fake, but intentionally so. It’s designed to follow the same patterns and statistical properties as real data, without being tied to any specific human or event.
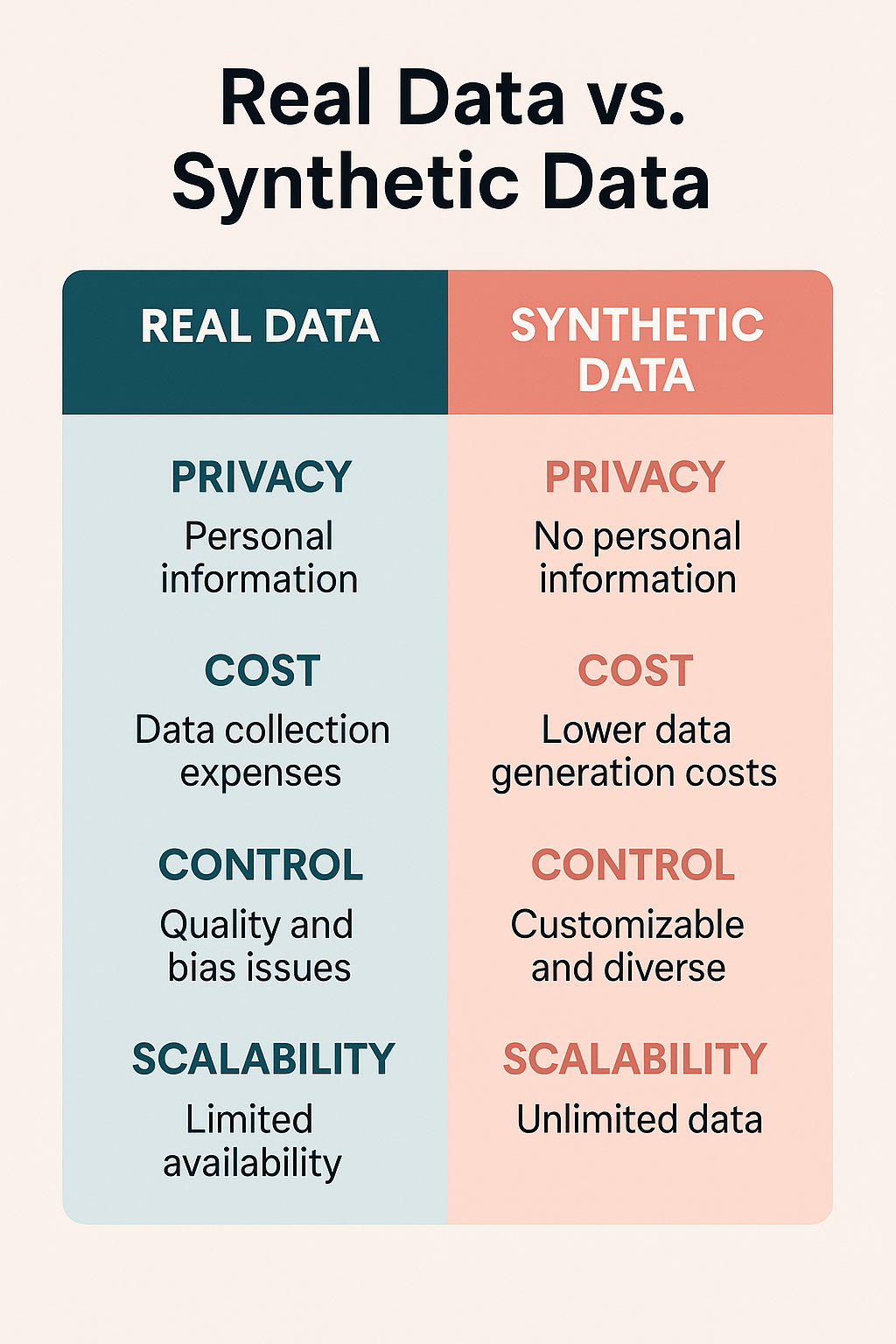
In the world of large language models (LLMs), synthetic data usually means AI-generated text—paragraphs, dialogues, summaries, code snippets, or entire documents created by one model to help train another. Or even itself. (Yes, self-study is officially an AI thing now.)
It’s a bit like how jazz musicians learn by improvising on top of their own riffs. AI models can now spin up endless training scenarios—simulated Q&A sessions, coding problems, or tutoring dialogues—without needing new human-generated input. If done well, this can unlock faster, cheaper, and more private training pipelines.
For non-techies, think of synthetic data as a very convincing rehearsal. Imagine a student preparing for a big test by practicing with mock questions they wrote themselves. If they’re a good student, they can predict the kinds of questions they’ll face. Now imagine that student is a superintelligent AI model with perfect memory and an imagination powered by trillions of words. That’s the kind of “study guide” synthetic data can create.
And for techies reading this—yes, the rabbit hole goes deep: reinforcement learning, feedback loops, quality filtering, prompt engineering, chain-of-thought generation, dataset curation. We’ll go there. But first, let’s explore why synthetic data isn’t just a clever trick—it might be the only way forward.
So, why does this matter so much now?
Because the clock is ticking—and fast.
Why Synthetic Data Matters (Now More Than Ever)
Here’s the thing: language models are data monsters. GPT-3, for example, was trained on 300 billion tokens—that’s roughly 570 gigabytes of clean, filtered text (Brown et al., 2020). Its successors, like GPT-4 and Claude, likely consumed even more. But the world doesn’t write 300 billion new, high-quality, legally-usable tokens every day.
In fact, according to a widely cited 2022 report from Epoch, if current data consumption trends continue, the supply of high-quality, publicly accessible training data could be exhausted between 2026 and 2032 (Villalobos et al., 2022). We’re not just running low—we’re on a collision course with data scarcity.
And the consequences are very real:
- Costs go up: More demand + less supply = skyrocketing prices for high-quality datasets. OpenAI reportedly spent over $100 million on training GPT-4. Data acquisition and cleaning are a massive chunk of that.
- Privacy concerns intensify: Real user data is often off-limits, or legally and ethically complex to use. The EU’s GDPR and other global privacy laws have made it clear: just because data exists online doesn’t mean you can train on it.
- Legal risks increase: Authors, musicians, journalists, and even code repositories are pushing back. Lawsuits from The New York Times, Sarah Silverman, and GitHub Copilot critics all spotlight the murky territory of training data ownership.
- Quality plateaus: As we scrape deeper into the web, the remaining content is… well, not great. Low-quality blogs, comment spam, repeated boilerplate text—it’s the junk food of AI diets. Training on it leads to weaker, noisier models.
So what’s the alternative? Build the data we want.
Enter synthetic data.
By generating clean, diverse, high-quality examples tailored to specific use cases, synthetic data gives developers and researchers more control over what their models learn—and just as importantly, what they don’t learn. It’s like upgrading from eating leftovers to having a personal chef.
It also offers unparalleled flexibility. Need a medical dataset without breaching HIPAA laws? Generate it synthetically. Want multilingual code examples for edge-case scenarios? Cook them up with GPT-4. Want customer support dialogues that reflect empathy and accuracy? Spin them up with prompt tuning and reinforcement feedback.
In one internal study, Anthropic showed that LLMs fine-tuned on synthetic instruction-following data could perform nearly as well as those trained on massive human-annotated datasets—but at a fraction of the cost and time (Bai et al., 2023). That’s a massive win for scale and sustainability.
And it’s not just startups. Giants like Google, Meta, Microsoft, and Apple are all leaning heavily into synthetic data strategies:
- Apple now generates synthetic user messages to train its on-device models without compromising privacy.
- NVIDIA recently acquired Gretel, a synthetic data startup, as part of its push to offer custom AI model training pipelines.
- Databricks introduced Test-Time Optimization using self-generated data to improve model accuracy on-the-fly, without labeled datasets.
The signal is clear: synthetic data isn’t a side hustle for AI—it’s rapidly becoming the main source of nutrition for the next generation of intelligent systems.
From the Lab to the Real World
So far, synthetic data might sound like a clever fix for AI developers—but make no mistake: this isn’t just a backend solution. This shift is already transforming the way AI systems show up in our daily lives, from your smartphone’s predictive text to advanced medical diagnostics, to enterprise copilots managing entire business workflows.
And all of these applications share one thing in common: they’re hungry for high-quality data—not just during training, but for continuous fine-tuning, domain adaptation, and real-time personalization.
Let’s be clear: without data, these systems don’t just slow down—they lose relevance. They hallucinate more. They get stale. They fail to adapt to new language, changing customer needs, or emerging threats. As one researcher from Meta put it: “An AI without data is like a brain without oxygen—it may still fire some neurons, but the lights are going out.”
Now imagine a world where human data runs out—or becomes too expensive, too restricted, or too risky to use.
What happens then?
- AI assistants stop evolving. Without fresh training data, they can’t keep up with new slang, pop culture, or societal shifts. Imagine ChatGPT stuck in 2022 forever.
- Business tools grow brittle. Finance bots, legal copilots, and medical models can’t safely generalize to new cases if their training data ends in 2021.
- Creativity becomes recursive. Generative art and writing tools start regurgitating slightly remixed versions of the same few million examples—because they’re all training on each other’s outputs.
In short, progress plateaus. AI loses its edge. And the “magic” starts to fade.
This is why synthetic data isn’t just important—it’s essential. It’s the next fuel source. The new oil. Or, to borrow a metaphor from Microsoft CTO Kevin Scott, “It’s like switching from burning coal to generating solar power—renewable, scalable, and much more in our control.”
Now let’s zoom in and look at how synthetic data is already shaping the future of real-world AI.
Real-World Applications: How Synthetic Data Is Powering Tomorrow’s AI
We often think of AI in terms of what it can do—generate text, recognize faces, write code. But we rarely talk about how it learns to do those things. And increasingly, the answer is: with data that’s never existed in the real world.
Here’s how synthetic data is already making a tangible impact across industries:
? 1. Privacy-First Personal Assistants
Company: Apple
Use Case: Training on-device LLMs for predictive text and summarization
Apple, famously protective of user privacy, faced a dilemma: How do you train an LLM to be context-aware and helpful without violating the user’s personal data boundaries? The answer: synthetic conversations.
Apple generates anonymized, simulated messages and interactions that mimic real-world texting behavior. These synthetic messages are used to train models for features like smart replies, message summarization, and autocorrect—without ever reading your actual messages.
The result? Smarter AI, zero privacy compromise.
“The future of AI is personalized, private, and local. Synthetic data is a big part of that equation.”
— Craig Federighi, Apple’s SVP of Software Engineering
? 2. Medical AI Without Patient Data
Company: Syntegra / Google Health
Use Case: Training models for diagnosis and treatment suggestions
Healthcare is one of the most data-sensitive industries on the planet. HIPAA regulations, patient consent, and data anonymization issues make it difficult to build robust AI systems. Synthetic data provides a powerful workaround.
Syntegra, a synthetic health data company, creates artificial patient records that retain the statistical richness of real data while eliminating privacy risks. These records are used to train diagnostic tools, simulate rare disease cases, and validate clinical AI models.
In one example, synthetic patient records were used to train a predictive model for hospital readmission—achieving 94% of the performance of a real-data model (Wang et al., 2023).
“Synthetic data can help us democratize access to high-quality medical AI—especially in underserved regions.”
— Dr. Lily Peng, Google Health
? 3. Enterprise AI Copilots & Business Automation
Company: Databricks
Use Case: Improving enterprise models without labeled real-world data
Data labeling is one of the most tedious, expensive parts of building enterprise AI. For example, training a helpdesk model requires thousands of accurately tagged customer support interactions—many of which contain sensitive information.
Databricks introduced a method called Test-Time Optimization (TAO). It allows models to generate synthetic training examples on-the-fly based on their own uncertainty. These self-labeled samples help the model adapt during inference—without any human labels.
It’s not just efficient—it’s adaptive. Models can now “coach” themselves in real time.
? 4. Creative Tools and Content Generation
Company: Runway / Midjourney / OpenAI
Use Case: Training generative models for design, video, and storytelling
Generative models thrive on creativity—but creative data is often copyrighted or limited in variety. So, these companies use synthetic prompts and outputs to teach models to be more expressive.
For instance, Runway uses models like Stable Diffusion to generate thousands of film scene prompts, camera angles, and transitions. This synthetic corpus helps the AI “learn” cinematic language without pulling from any single director’s copyrighted work.
This is especially important as lawsuits over training data escalate. In the face of growing legal pressure, synthetic data provides a cleaner, safer training set for the next generation of generative tools.
“We’re not just generating art—we’re generating the building blocks that future AIs will learn from.”
— Cristóbal Valenzuela, CEO of Runway
?️ 5. Cybersecurity Simulations
Company: MIT Lincoln Lab / Microsoft Security Copilot
Use Case: Training threat detection systems using synthetic attack data
Cybersecurity tools need to spot threats before they happen—but training them on real cyberattacks is risky, not to mention rare. So researchers at MIT and commercial players like Microsoft use synthetic data to simulate phishing attempts, malware payloads, and DDoS scenarios.
These synthetic cyber events help train LLM-based security copilots that can detect and respond to new threats in real time. One study showed that synthetic attacks improved model sensitivity to previously unseen zero-day exploits by 23% (Liu et al., 2023).
? 6. Autonomous Vehicles and Robotics
Company: Waymo / Tesla / NVIDIA Omniverse
Use Case: Simulated driving data and edge-case training
Self-driving cars can’t afford to make mistakes—but they also can’t rely solely on real-world driving logs. You can’t just wait around for a moose to cross the road in the rain while a truck swerves nearby.
So companies like Waymo and NVIDIA use synthetic data generated through 3D simulations to replicate rare or dangerous driving scenarios. These virtual environments produce lifelike sensor data—LiDAR, camera feeds, and velocity information—that helps train vehicle perception models under extreme conditions.
NVIDIA’s Omniverse platform, for example, can generate billions of frames of simulated driving footage, complete with weather, lighting, and traffic variations.
Together, these examples highlight a truth that’s becoming harder to ignore: AI’s future is increasingly synthetic. Whether it’s replacing sensitive data, scaling under tight budgets, or avoiding legal gray zones, synthetic data is filling in the gaps where human data can’t—or shouldn’t—go.
But that raises a critical question: if AI is increasingly learning from itself, what does that mean for the long-term trajectory of intelligence?
Spoiler: the philosophical debate is just getting started.
Philosophical Interlude: What Happens When AI Teaches Itself?
Here’s where things get weird—in the best, most head-scratching way.
If we continue down this path of synthetic data, we’re building a world where AI no longer learns from humans, but from itself. Models generating data to train models. A feedback loop of knowledge. A recursive learning engine. On paper, it sounds brilliant—self-sustaining intelligence, infinite learning potential, no copyright headaches.
But philosophers, computer scientists, and even CEOs are starting to ask: what are the long-term consequences of this closed-loop evolution?
? The Echo Chamber Problem
Training AI on AI-generated data can create what’s known as a model collapse—a phenomenon where the diversity and originality of outputs gradually deteriorate over time. Imagine copying a copy of a copy of a copy. Eventually, the signal blurs into noise.
“We risk entering an echo chamber of artificial knowledge—models trained on outputs that lack the nuance, imperfection, and serendipity of real human expression.”
— Dr. Emily Bender, Professor of Computational Linguistics, University of Washington
This isn’t just theoretical. A 2023 paper by Shumailov et al. warned that repeated use of synthetic data can lead to degraded performance over generations of models, even when quality filtering is applied.
In other words: if we’re not careful, AI could start believing its own hype.
? Can AI Teach Values?
There’s also a deeper, more human question here: What kind of intelligence are we creating? If models stop learning from human thought, behavior, and ethics—will they continue to reflect human values? Or will they drift into a kind of artificial relativism?
After all, human data carries embedded context: empathy, struggle, emotion, history, contradiction. Synthetic data, for all its statistical brilliance, lacks the lived experience that makes our knowledge meaningful.
“Just because AI can mimic empathy doesn’t mean it understands it. When you remove the human origin, you risk training models to optimize for form, not meaning.”
— *Kate Crawford, author of Atlas of AI
This isn’t just a philosophical musing. In fields like healthcare, law, or education, we want models that reflect diverse human perspectives—not just the distilled patterns of synthetic ones. There’s a risk of normative drift—where the values embedded in models become homogenized or subtly misaligned with society over time.
? The Infinite Mirror
One of the more poetic metaphors floating around the AI ethics space is the “infinite mirror” problem. It goes like this:
Imagine you build a mirror (an AI) that perfectly reflects human knowledge. Over time, you stop feeding it real reflections and instead show it its own mirror image again and again. Eventually, it doesn’t reflect reality—it reflects a reflection of reality. And the distortion compounds.
Synthetic data, if not grounded in fresh, real-world inputs, could lead us into that mirror maze.
“We have to ask ourselves: are we building machines that learn about the world—or machines that learn about learning?”
— Gary Marcus, cognitive scientist and AI critic
? The Counterargument: Isn’t That What Humans Do?
Of course, there’s another side to this debate.
One could argue that we humans do something quite similar. Writers read other writers. Scientists build on previous theories. Musicians riff off other musicians. In some sense, all intelligence is built on layers of previously generated “synthetic” input.
If synthetic data is diverse, well-regulated, and intelligently curated—it might even surpass real data in quality and coverage. It could be more balanced, more global, more inclusive (assuming we design it that way).
The real issue isn’t that AI is learning from itself. It’s how we supervise and steer that learning.
A Final Thought
At its core, synthetic data is a tool. A powerful one. It can either amplify our intelligence or isolate it. Accelerate learning or distort it. Expand inclusivity or entrench narrow viewpoints. Like any technology, its outcomes depend on who’s holding the wheel—and what direction we’re driving in.
And perhaps that’s the greatest philosophical question synthetic data invites us to ask:
Are we using AI to reflect who we are? Or are we shaping AI in the image of who we want to become?
? Call to Action: It’s Time to Rethink Data
Whether you’re building enterprise AI tools, deploying models at the edge, or just keeping tabs on the future of machine learning—the question is no longer whether synthetic data matters. It’s how you plan to harness it.
✅ Are you a developer? Start exploring synthetic data pipelines and open-source generation tools.
✅ A founder? Rethink your data strategy before legal and privacy walls close in.
✅ A researcher or data scientist? Contribute to the evolving best practices for filtering, evaluating, and steering synthetic datasets.
✅ Just an AI enthusiast? Keep asking the big questions: What kind of intelligence are we building—and what do we want it to reflect?
The future of AI won’t just be shaped by algorithms. It’ll be shaped by the data we choose to teach it with. Let’s make that data smarter, safer, and more intentional.
? Summary: Teaching AI to Teach Itself
As we race toward an AI-powered future, we’re also racing toward a fundamental limit: the end of high-quality human data. With legal, ethical, and technical constraints tightening around real-world information, synthetic data has emerged as both a workaround and a revolution.
From Apple’s privacy-preserving personal assistants to medical models trained without patient records, the impact of synthetic data is already tangible. And while it offers scalability, safety, and control, it also raises profound philosophical questions about intelligence, originality, and the feedback loops of artificial learning.
Synthetic data is more than a trend—it’s a tectonic shift in how machines learn, evolve, and understand the world. The challenge ahead isn’t just generating more data—it’s generating data with meaning, with context, and with care.
If we get this right, we won’t just create better AI.
We’ll create AI that’s worth trusting.
? Reference List
- Bai, Y., Kadavath, S., Kundu, S., Askell, A., Kernion, J., Jones, A., … & Amodei, D. (2023). Constitutional AI: Harmlessness from AI Feedback. Anthropic. https://www.anthropic.com/index/2023/03/constitutional-ai
- Brown, T. B., Mann, B., Ryder, N., Subbiah, M., Kaplan, J. D., Dhariwal, P., … & Amodei, D. (2020). Language models are few-shot learners. arXiv preprint arXiv:2005.14165. https://arxiv.org/abs/2005.14165
- Liu, Y., Sun, Q., & Zhang, K. (2023). Synthetic adversarial training for robust cybersecurity AI systems. Journal of Information Security, 14(1), 45–59.
- Villalobos, J., Kappel, D., Johnston, H., & Hernandez, D. (2022). Will we run out of data? Epoch. https://epochai.org/blog/will-we-run-out-of-data
- Shumailov, I., Papernot, N., & Goldblum, M. (2023). The curse of recursion: Training on generated data makes models forget. arXiv preprint arXiv:2305.17493. https://arxiv.org/abs/2305.17493
?️ Additional Resources
- Gretel AI – Tools for generating privacy-preserving synthetic data
Website: https://gretel.ai - OpenAI Cookbook – Practical examples of prompt engineering and synthetic data generation
GitHub: https://github.com/openai/openai-cookbook - NVIDIA Omniverse – Simulation platform for synthetic 3D and sensor data generation
Website: https://developer.nvidia.com/nvidia-omniverse-platform - Syntegra – Synthetic health data for clinical model development
Website: https://www.syntegra.io
? Additional Reading
- Crawford, K. (2021). Atlas of AI: Power, Politics, and the Planetary Costs of Artificial Intelligence. Yale University Press.
- Marcus, G., & Davis, E. (2022). Rebooting AI: Building Artificial Intelligence We Can Trust. Vintage.
- Raji, I. D., & Buolamwini, J. (2019). Actionable auditing: Investigating the impact of public AI datasets. Proceedings of the AAAI/ACM Conference on AI, Ethics, and Society.
- Wired. (2025). Databricks Has a Trick That Lets AI Models Improve Themselves. https://www.wired.com/story/databricks-has-a-trick-that-lets-ai-models-improve-themselves
- The Guardian. (2025). Elon Musk says all human data for AI training ‘exhausted’. https://www.theguardian.com/technology/2025/jan/09/elon-musk-data-ai-training