Disclaimer
This content is for informational purposes only and is not a substitute for professional medical advice, diagnosis, or treatment. Always consult a qualified healthcare provider with any health-related questions or concerns.
Introduction: The Evolution of Breast Cancer Screening and the Promise of AI
Breast cancer has long been a formidable adversary in women’s health, with early detection being a critical factor in improving survival rates. Historically, the journey of breast cancer screening has evolved from rudimentary clinical examinations to sophisticated imaging techniques, culminating in the integration of artificial intelligence (AI) to enhance diagnostic accuracy.
The Pre-AI Era: Foundations of Breast Cancer Screening
In the early 20th century, breast cancer detection primarily relied on physical examinations, which often identified tumors at advanced stages, limiting treatment options and reducing survival rates. The introduction of mammography in the 1960s marked a significant advancement, allowing for the visualization of breast tissue and the identification of tumors before they became palpable. Pioneering studies, such as those led by Dr. Philip Strax, demonstrated that regular mammographic screening could reduce breast cancer mortality by detecting cancers earlier (Strax et al., 1971).
Despite these advancements, traditional mammography has limitations, including false positives, false negatives, and reduced sensitivity in women with dense breast tissue. These challenges underscored the need for more precise diagnostic tools to improve early detection and reduce unnecessary interventions.
The Importance of Early Detection
Early detection of breast cancer significantly enhances treatment outcomes. According to the American Cancer Society, when breast cancer is detected at a localized stage, the 5-year relative survival rate is approximately 99% (American Cancer Society, 2023). Early-stage detection often allows for less aggressive treatments, preserving quality of life and reducing healthcare costs.
The Advent of AI in Breast Cancer Screening
The integration of AI into breast cancer screening represents a transformative shift in diagnostic methodologies. AI algorithms, particularly those utilizing deep learning, can analyze vast datasets of mammographic images to identify patterns indicative of malignancy with remarkable accuracy.
Recent studies have highlighted the efficacy of AI in improving diagnostic precision. For instance, a study published in JAMA Network Open demonstrated that an AI algorithm could predict the risk of future breast cancer development, enabling proactive monitoring and intervention (Yala et al., 2024).
Revolutionizing the Screening Process
AI’s ability to process and interpret complex imaging data enhances the detection of subtle anomalies that may be overlooked by human observers. This capability is particularly beneficial in cases involving dense breast tissue, where traditional mammography’s sensitivity is diminished. By augmenting radiologists’ assessments, AI contributes to more accurate diagnoses and personalized patient care.
A Philosophical Perspective: Balancing Technology and Human Touch
The integration of AI into healthcare prompts philosophical considerations regarding the balance between technological advancement and the human elements of medical practice. While AI offers unparalleled analytical capabilities, the empathetic communication and nuanced judgment of healthcare professionals remain irreplaceable. The synergy between AI and clinicians can lead to a more holistic approach to patient care, combining data-driven insights with compassionate support.
Summary
The evolution of breast cancer screening, from manual examinations to AI-enhanced diagnostics, reflects the medical community’s commitment to improving patient outcomes through innovation. By embracing AI’s potential while preserving the essential human aspects of care, we can aspire to a future where breast cancer is detected earlier, treated more effectively, and, ultimately, overcome.
Yet, even with all the progress made through decades of research and improved imaging, challenges remain. False positives still cause anxiety and lead to unnecessary procedures. Dense breast tissue continues to obscure critical findings. And overburdened radiologists, working under time pressure, face increasing workloads as screening programs expand worldwide.
So, what if there was a way to reduce those burdens? To see what the human eye might miss? To personalize risk assessment based on each woman’s unique data—not just her age or family history?
That’s where artificial intelligence steps in—not to replace the doctor, but to become their most insightful second opinion.
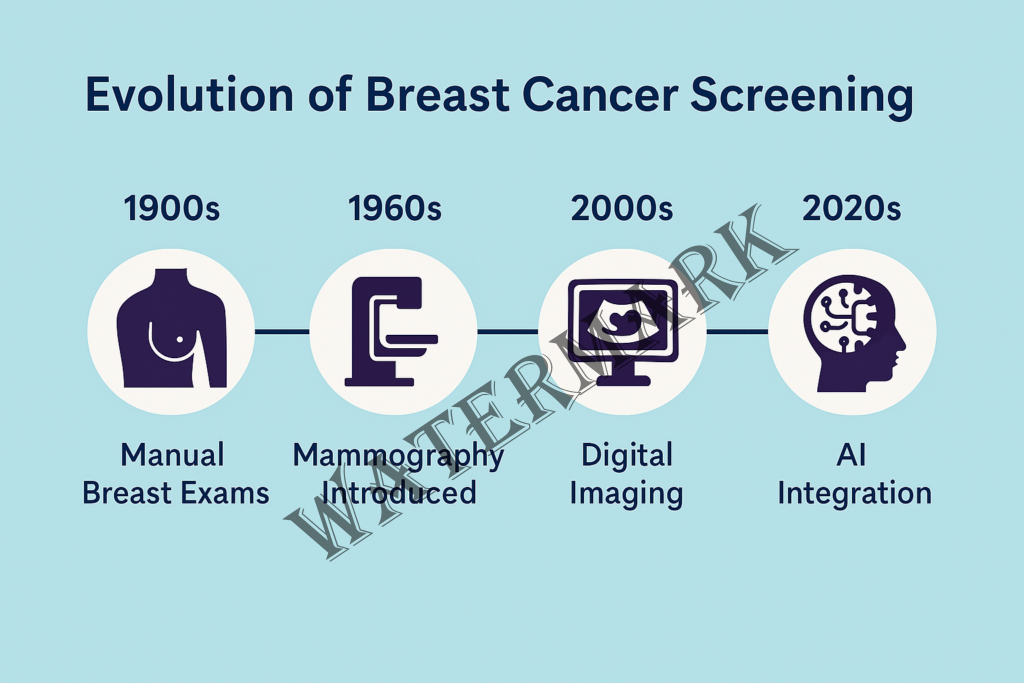
The Promise of AI in Mammography
Artificial intelligence in mammography might sound futuristic, but it’s no longer a concept—it’s here, it’s tested, and in many places, it’s already saving lives.
AI’s role isn’t to replace radiologists; it’s to stand beside them, acting like a super-charged assistant that never gets tired, never misses a pixel, and can spot patterns invisible to even the most experienced human eyes. At its core, AI in mammography is about boosting accuracy, reducing errors, and catching cancers earlier—when they’re most treatable.
But let’s back up a bit. Has it been tested? Oh yes.
One of the biggest breakthroughs came from a large-scale clinical trial in Sweden known as the MASAI study. Researchers used AI to analyze mammograms from over 80,000 women. The result? AI-supported screening detected 20% more cancers than traditional methods, without increasing the number of false positives (Lång et al., 2025). Even more impressively, the workload for radiologists was reduced by nearly half. Imagine what that could mean for overworked healthcare systems around the world.
Another standout example comes from the MIT Jameel Clinic, where researchers developed a deep learning model capable of predicting breast cancer risk up to five years in advance (Yala et al., 2024). This is a game-changer—because instead of just reacting to suspicious lumps or shadows, doctors can begin proactively monitoring patients who are at high risk before tumors even form.
But of course, it hasn’t all been smooth sailing.
In its early days, AI in radiology had to battle skepticism. Some systems overfit to the data they were trained on—meaning they performed well in test environments but floundered in real-world clinical settings. Others were trained on datasets that lacked diversity, raising concerns about racial and ethnic bias. In some studies, early AI models performed worse for Black women and women with dense breast tissue, potentially widening existing healthcare disparities.
These issues weren’t just technical—they were ethical. But to the credit of the research community, these concerns didn’t go ignored. Developers began improving data transparency, diversifying training datasets, and building “explainable AI” models that radiologists could trust and understand, rather than blindly follow. The technology learned. The teams adapted. And the models improved.
Today, AI is no longer just a proof-of-concept. It’s being used in hospitals from Germany to California—and even in low-resource settings where radiologists are in short supply.
Which brings us to a big question: how can we implement this at scale, and do it affordably?
Because let’s face it, a technology that only helps the few isn’t really solving a global problem.
Here’s where thoughtful implementation matters. Some experts suggest a hybrid approach: using AI as a first-read tool that flags suspicious cases for radiologists to review more carefully. This method has already shown promise in places like the UK and Sweden, where it’s helping reduce diagnostic delays without fully replacing human oversight. It’s cost-effective and scalable—especially in public health systems.
Then there’s the question of access. Could AI eventually help clinics in rural or underserved areas? Possibly. Instead of flying in radiologists once a month or mailing mammograms out to cities for review, AI tools could provide instant, on-site analysis—saving time, money, and lives.
Still, insurance coverage and regulatory approvals will play a big role. In the U.S., AI-based screenings aren’t always reimbursed yet, which can be a barrier to adoption. Advocacy from medical professionals, patient groups, and tech leaders is helping to push that conversation forward. Regina Barzilay, an MIT professor and breast cancer survivor herself, has been a vocal advocate, noting that “technology shouldn’t just advance—it should reach everyone.”
As AI continues to prove itself in labs and clinics, the excitement isn’t just academic. It’s personal. It’s professional. And it’s emotional.
Behind every algorithm and clinical trial, there are people—doctors, researchers, patients—who are championing this movement not just because it’s innovative, but because it matters. These voices remind us that while AI may be powered by data, it’s driven by human stories.
Voices from the Field: Where Passion Meets Precision
Dr. Kristina Lång – The Trailblazer
If there’s one person at the front lines of AI in breast imaging, it’s Dr. Kristina Lång, lead researcher of the groundbreaking MASAI trial in Sweden.
Poised, pragmatic, and quietly revolutionary, Dr. Lång didn’t just run a trial—she opened a door to a new era. When asked about the results of the study, she doesn’t focus on the tech specs. Instead, she zeroes in on what matters most:
“AI-supported screening can significantly enhance the early detection of clinically relevant breast cancers,” she said in an interview. “But equally important—it can help radiologists focus on the cases that need their expertise most.” (Lång et al., 2025)
There’s a spark in how she talks about AI: not as a replacement, but as an evolution. A way to make mammography smarter and more human at the same time.
Regina Barzilay – The Survivor Turned Scientist
Then there’s Regina Barzilay—a name you’ll hear often in AI healthcare circles. She’s a professor at MIT, a MacArthur “genius” grant recipient, and one of the most influential voices in AI research.
But what makes her story hit hard is what came before the accolades. Barzilay is a breast cancer survivor. When she was diagnosed, she began asking a different kind of question: why weren’t we using machine learning to predict this earlier?
Instead of waiting for someone else to solve the problem, she helped build the solution.
“The experience of being a patient changes everything,” she said during a talk at MIT. “You stop seeing the gaps in care as theoretical. You live them. And that gives you urgency.”
Her work now helps train AI models to identify patterns that signal a high risk of cancer—even years before a tumor forms. And while she’s deeply technical, she’s equally philosophical:
“I don’t want AI to just be smarter than us. I want it to be wiser with us.”
Dr. Constance Lehman – The Connector
At Massachusetts General Hospital, Dr. Constance Lehman serves as both a radiologist and a connector between medicine and machine learning. She’s one of the voices pushing for AI to not just be clinically effective—but equitably deployed.
She’s especially focused on the problem of access—on making sure rural clinics and underserved communities aren’t left behind.
“If AI helps only patients who live near elite hospitals, then we’ve missed the point,” she says. “The goal is not to create smarter machines. The goal is to create a smarter system.”
Her team has been exploring hybrid AI models where clinicians in remote areas use AI as a triage tool—identifying patients who need urgent follow-up. It’s not about cutting corners—it’s about widening the circle of care.
The Radiologist Who Hesitated—Then Believed
Not every AI champion started out as one. Dr. Anand Patel, a radiologist in California, was one of many professionals who greeted AI with skepticism.
“My first thought was: Great, another Silicon Valley solution looking to disrupt a field they don’t understand.”
But after using AI in clinical workflows for a year, his tune changed.
“It caught two early-stage cancers that I almost missed. I still think about that. It humbled me—and it convinced me. This isn’t a threat. It’s a teammate.”
These voices remind us that AI in breast cancer screening isn’t just a technology story. It’s a human story.
It’s doctors wanting more time with their patients. It’s survivors asking bigger questions. It’s researchers pushing for a future that’s not just high-tech, but high-trust.
And as we listen to these voices, we realize something important: the heart of this innovation isn’t the code—it’s the people it empowers.
Listening to these passionate voices—from seasoned clinicians to survivor-scientists—it becomes clear that AI in breast cancer detection isn’t just a technological shift. It’s a cultural one. It forces us to ask not only what AI can do, but also what it should do.
Because the real revolution here isn’t only in lines of code—it’s in how we redefine trust, responsibility, and even the role of the physician.
This brings us into the realm of philosophy, where ethical questions, human values, and technology intersect. And where medicine’s future will be shaped not just by engineers, but by the collective soul of society.
Philosophical Reflections: What Happens When Machines Start to See What We Can’t?
1. Trust and Transparency – Can We Trust a “Black Box”?
One of the core tensions in AI-assisted care is trust. Many deep learning models are famously opaque—what’s often called the “black box” problem. They deliver results, but can’t always explain how they got there in a way a clinician can confidently interpret.
That might be acceptable when AI recommends a movie. But when it flags an early-stage cancer? The stakes are profoundly different.
As Dr. Anita Goyal, an AI ethicist at Stanford, puts it: “Patients deserve to know whether the decisions made about their bodies come from human experience or an algorithm trained on 50 million data points.”
This leads to a growing push for explainable AI (XAI)—systems that not only perform well but communicate how they arrived at their conclusions. It’s not just about better diagnostics; it’s about respecting patient autonomy and clinician confidence.
2. Empathy and the Human Element – What About the Bedside Manner?
Can AI offer comfort? Can it understand fear?
Of course not. That’s the irreplaceable realm of human care.
While AI may read a scan with superhuman precision, it cannot hold a patient’s hand after a diagnosis, or intuit when someone needs more than information—they need reassurance.
There’s a growing philosophical concern that medicine could become too transactional if we over-rely on AI: all outputs and inputs, no emotion. But the best implementations of AI don’t remove the human touch—they amplify it. By taking over repetitive tasks, AI can give doctors more time to connect, listen, and support.
In this way, AI can actually help restore the art of medicine that’s so often lost in the paperwork and pace.
3. Responsibility and Accountability – Who Gets the Blame?
Imagine a scenario where an AI system misses a tumor—or worse, gives a false positive that leads to an unnecessary biopsy. Who is responsible? The developer? The hospital? The radiologist who trusted the tool?
These are not just hypotheticals. They’re already making their way into legal and ethical debates across healthcare systems worldwide.
The deeper issue here is: how do we share responsibility between man and machine? AI doesn’t have moral agency. It can’t be sued or shamed. Yet its decisions can alter lives.
Professor Ezekiel Emanuel writes, “We must stop thinking of AI as a tool and start seeing it as a partner—one with power, and therefore, with shared accountability.”
Some suggest a “human-in-the-loop” model, where AI assists but does not make final decisions. Others push for regulatory frameworks that demand transparency and documentation for every AI-driven decision. Either way, responsibility can’t vanish into the cloud.
4. Bias and Equity – Can AI Be Fair?
AI is only as good as the data it’s trained on. If that data underrepresents certain populations—say, women of color, transgender patients, or individuals with rare genetic backgrounds—then the model may perpetuate those blind spots.
This raises deep ethical questions about equity in medicine. Is AI leveling the playing field—or reinforcing the gaps?
The good news is that awareness around this is growing. Developers are working to diversify training data, and institutions are beginning to test AI tools across demographics before implementation. But it’s not enough to aim for “accuracy”—we must also strive for justice in healthcare AI.
5. Consent and Autonomy – Do Patients Know AI Is Involved?
Many patients assume their care is being handled by human professionals. But increasingly, AI is behind the scenes—analyzing images, flagging concerns, predicting risk. And often, patients don’t even know.
This opens a philosophical can of worms. If patients aren’t told an AI helped inform their care, are they truly giving informed consent? Should they be?
Some argue it’s no different than using advanced software or machines in surgery. Others contend that as AI takes on more diagnostic responsibility, transparency must follow. Patients have a right to know not only what is happening to their bodies, but who—or what—is helping make those calls.
6. The Nature of Expertise – Are We Redefining the Role of the Doctor?
AI’s rise challenges a deeply ingrained idea: that the doctor is the sole authority in the exam room.
But if an algorithm can predict cancer better than a trained radiologist, what does that mean for the physician’s role? Are we transitioning from doctor-as-diagnostician to doctor-as-decision-coach?
It’s a profound shift—and one that may initially feel like a loss of identity for some medical professionals. But others see it as a liberation: a chance to return to what many doctors went into medicine for in the first place—to care, to connect, and to guide.
As one radiologist said in a panel discussion: “AI won’t replace me. But it might replace the version of me that’s buried under 10 hours of image analysis.”
So, What Do We Make of All This?
The future of breast cancer detection is not just about faster scans or better algorithms—it’s about navigating a new relationship between humans and machines. It’s about choosing to design that relationship thoughtfully.
Technology, after all, is not inherently good or bad—it reflects the values of those who build and use it. And when it comes to health, those values must be deeply human.
If AI forces us to rethink what it means to diagnose, to trust, and to care—then the next logical question is this:
How do we actually make it work in the real world?
Because no matter how promising the algorithms or how elegant the philosophy, the success of AI in breast cancer detection will ultimately be judged by what happens in hospitals, clinics, and communities. Not in theory—but in practice.
It’s easy to get swept up in headlines about “AI outperforming doctors.” But when the cameras leave and the tech buzz fades, what’s left is something far more complex: budgets, logistics, human behavior, insurance codes, rural clinics, cultural norms, and regulatory red tape.
So let’s bring this back to earth.
Challenges and Considerations: Bridging the Gap Between Innovation and Implementation
1. The Cost Question – Who Pays for Intelligence?
AI might be “smart,” but it’s not free. High-performance AI systems require powerful computing infrastructure, software licenses, integration into clinical workflows, and—most crucially—training for the humans who use them.
While elite hospitals may be able to afford these costs, smaller clinics, particularly in rural or underserved areas, may not.
A recent analysis found that while AI improved detection rates, the lack of insurance reimbursement for AI-assisted screenings made widespread adoption financially unsustainable for many providers (Popsugar, 2025).
Here’s the paradox: the places that might benefit most from AI—understaffed, resource-strapped facilities—are the least likely to afford it.
That’s why many advocates are calling for public-private partnerships, government subsidies, and tiered pricing models to ensure that access to better care isn’t just a perk for the privileged.
2. The Workflow Problem – How Do You Plug In a Paradigm Shift?
Imagine giving radiologists a Ferrari engine—but bolting it onto a bicycle frame.
That’s what AI can feel like if it’s not seamlessly integrated into clinical workflows. Some early adopters have struggled with clunky interfaces, redundant steps, and alert fatigue—where too many AI “flags” overwhelm rather than assist.
The most effective implementations are deeply collaborative, involving both clinicians and developers from the start. It’s not just about performance; it’s about fit. Does the AI tool work within the rhythm of a real clinic? Does it speed up the process—or slow it down with friction?
As one radiology tech put it, “If it’s just one more dashboard we have to check, no one will use it.”
3. The Training Gap – Who’s Teaching the Teachers?
Another overlooked challenge is education. AI can only be effective if the people using it understand how it works—and how it fails.
Clinicians don’t need to become data scientists, but they do need to know how to interpret AI outputs, question suspicious results, and recognize algorithmic blind spots.
A 2024 study found that nearly 60% of radiologists surveyed felt “unequipped” to work confidently with AI tools (Ghasemi et al., 2024).
The solution? Invest in cross-disciplinary training—bringing together medicine and machine learning not just at the tool level, but at the human level.
4. Regulation and Liability – Who’s On the Hook?
We touched on this in the philosophical section, but let’s get practical: right now, regulatory frameworks for AI in healthcare are playing catch-up.
In many countries, AI tools are approved under software-as-a-medical-device (SaMD) classifications, but there’s little consistency around monitoring, reporting failures, or defining liability when things go wrong.
Until the rules are clear, many hospitals are hesitant to fully trust AI tools—not because they doubt the science, but because they fear the legal fallout.
As AI becomes more autonomous, expect a surge in legislation, new FDA guidelines, and professional standards defining how AI fits into the chain of clinical accountability.
5. Cultural Resistance – The Human Side of Adoption
Let’s be real: some doctors just don’t want AI in their reading rooms.
Not because they’re technophobic. But because they’re tired of hype cycles. They’ve seen tools come and go—each one promising to revolutionize care, and many quietly shelved when they didn’t deliver.
“Show me the evidence,” one clinician said during a conference Q&A. “Don’t show me the TED Talk.”
To earn widespread adoption, AI must not only prove itself statistically—it must win hearts and minds. That means respecting clinical expertise, offering clear benefits, and being humble enough to listen to frontline feedback.
Because in the end, even the smartest tool is useless if no one wants to use it.
So… What Does Success Actually Look Like?
Success in AI-driven breast cancer screening isn’t measured only in detection rates or algorithm performance.
It looks like:
- A rural clinic catching an aggressive tumor that would’ve otherwise been missed.
- A radiologist having more time to counsel a worried patient instead of scanning backlogs.
- A patient in a public hospital receiving the same quality of care as someone in a top-tier private facility.
- A system that values both precision and empathy.
Success is when technology bends to meet human needs—not the other way around.
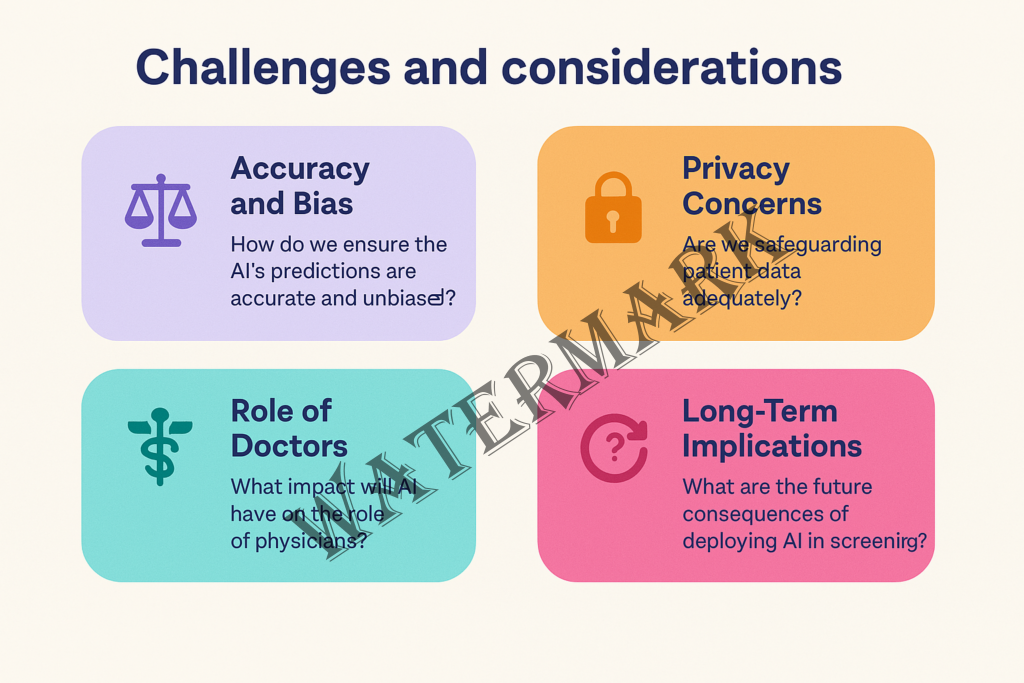
A Moment of Choice: Your Role in the Future of Care
As we stand at this pivotal intersection of medicine and machine learning, one truth becomes clear: the future of breast cancer detection doesn’t belong to AI alone—it belongs to all of us.
Whether you’re a clinician, a developer, a policymaker, a patient—or just someone who loves someone at risk—you have a role to play.
You can ask harder questions. Push for transparency. Support access and equity. Challenge the systems that decide who gets innovation and who gets left behind.
Because the success of AI in healthcare won’t be written in code. It will be written in values.
So here’s the call:
Let’s be thoughtful. Let’s be bold.
Let’s build a future where no one is missed, no matter where they live or what they look like.
Let’s make sure this isn’t just a technological leap—but a moral one.
Conclusion: From Innovation to Impact
Artificial intelligence is not the hero of this story. We are.
AI is the tool. A brilliant one. But like any tool, it depends on the hands—and the hearts—that wield it.
We began with the story of breast cancer screening—how it evolved from touch, to imaging, to intelligence. We explored the successes, the growing pains, the philosophical debates, and the real-world complexities. And through it all, one thing remains constant:
Human care, guided by human values, is what truly saves lives.
So as this new era unfolds, let’s lead it with curiosity. With compassion. With courage.
Because early detection is powerful.
But early transformation—that’s revolutionary.
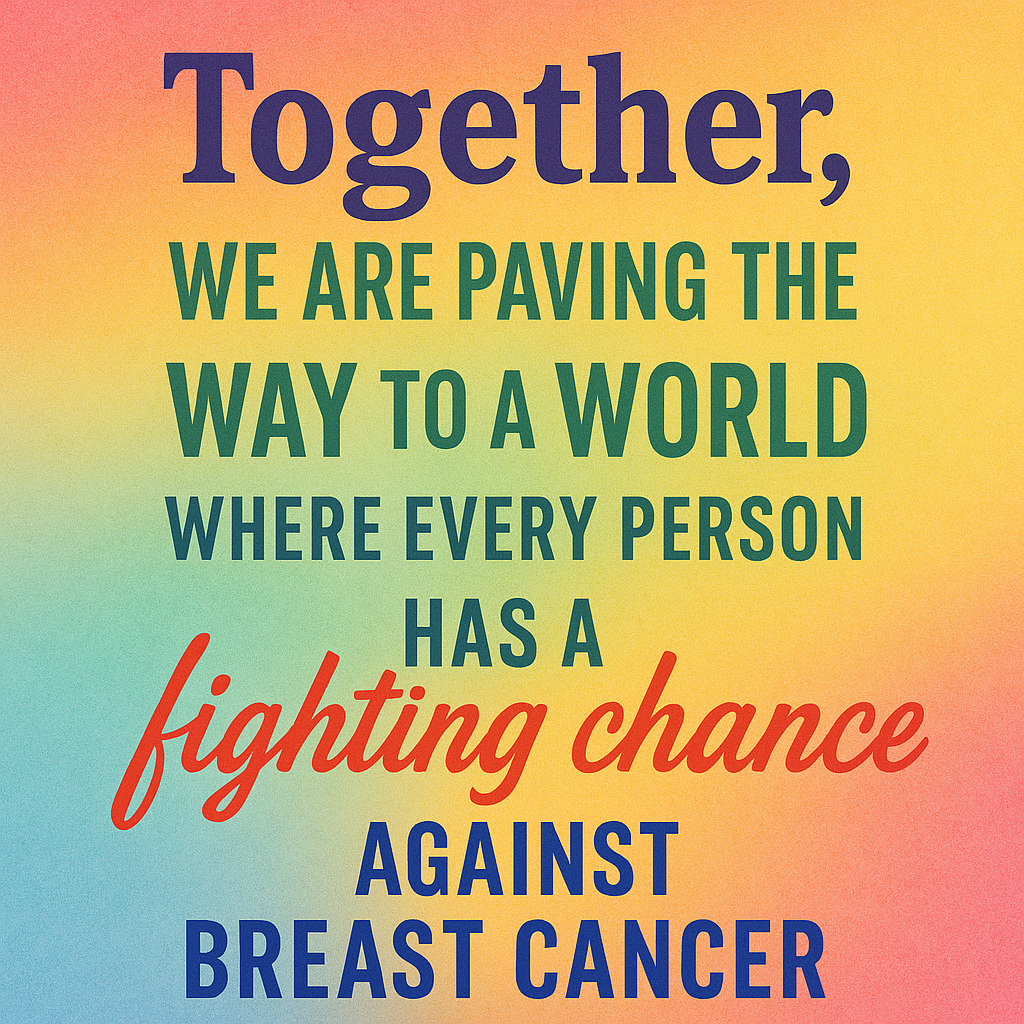
References
- American Cancer Society. (2023). Breast Cancer Facts & Figures 2023–2024. https://www.cancer.org/research/cancer-facts-statistics.html
- Bergtholdt, H., et al. (2025). Nationwide real-world implementation of AI for cancer detection in mammography screening. Nature Medicine. https://www.nature.com/articles/s41591-024-03408-6
- Ghasemi, A., Hashtarkhani, S., Schwartz, D. L., & Shaban-Nejad, A. (2024). Explainable artificial intelligence in breast cancer detection and risk prediction: A systematic scoping review. arXiv preprint arXiv:2407.12058. https://arxiv.org/abs/2407.12058
- Lång, K., et al. (2025). AI-enhanced mammography screening significantly improves breast cancer detection and reduces radiologist workload. The Lancet Digital Health. https://www.thelancet.com/journals/landig/article/PIIS2589-7500(24)00267-X/fulltext
- Popsugar. (2025). Getting a Mammogram? AI May Be Analyzing Your Breasts. https://www.popsugar.com/health/ai-mammograms-explained-49357278
- Strax, P., Venet, L., & Shapiro, S. (1971). Periodic breast cancer screening in reducing mortality from breast cancer. JAMA, 215(11), 1777–1785.
- Yala, A., et al. (2024). Artificial Intelligence Algorithm for Subclinical Breast Cancer Detection. JAMA Network Open, 7(1), e242345. https://jamanetwork.com/journals/jamanetworkopen/fullarticle/2824353
Additional Readings
- Ahmed, M., Bibi, T., Khan, R. A., & Nasir, S. (2024). Enhancing Breast Cancer Diagnosis in Mammography: Evaluation and Integration of Convolutional Neural Networks and Explainable AI. arXiv. https://arxiv.org/abs/2404.03892
- Maistry, B., & Ezugwu, A. E. (2023). Breast Cancer Detection and Diagnosis: A Comparative Study of State-of-the-Arts Deep Learning Architectures. arXiv. https://arxiv.org/abs/2305.19937
- National Cancer Institute. (2024). Artificial Intelligence in Cancer Research and Care. https://www.cancer.gov/research/areas/technology/artificial-intelligence
Additional Resources
- BreastCancer.org – Trusted patient education and research updates: https://www.breastcancer.org
- MIT Jameel Clinic – Leading research in AI and healthcare: https://jameelclinic.mit.edu
- American College of Radiology (AI in Breast Imaging) – Clinical guidelines and updates: https://www.acr.org/Clinical-Resources/Breast-Imaging
- Radiological Society of North America (RSNA) – Resources on AI in radiology: https://www.rsna.org/en/news/2023/October/AI-in-Breast-Imaging
Support the Cause – Trusted Donation Links
If this post moved you and you’re wondering how to help, consider making a donation to support breast cancer awareness, research, and access to care:
- Susan G. Komen Foundation
https://www.komen.org
(Focus: Research, education, and community outreach) - Breast Cancer Research Foundation (BCRF)
https://www.bcrf.org
(Focus: Funding world-class research for prevention and a cure) - National Breast Cancer Foundation
https://www.nationalbreastcancer.org
(Focus: Patient education, early detection, and screenings for underserved communities) - The Pink Fund
https://www.pinkfund.org
(Focus: Providing financial support to breast cancer patients in treatment)