Introduction: “The Calm Before the Chaos”
The energy at InnovaTech headquarters buzzed louder than the fluorescent lights overhead.
It was T-minus three days to launch — and everything, at least on the surface, was finally falling into place. The product, a sleek AI-powered project management tool, had been two years (and countless coffee runs) in the making. Investors were leaning in. Industry blogs were teasing a “disruptor to watch.”
Even the notoriously skeptical beta testers had issued cautious thumbs-up emojis in Slack.
Inside the war room — a glass-walled conference room now buried under pizza boxes, whiteboard scribbles, and half-sipped energy drinks — the team was giddy. Exhausted, yes, but genuinely proud. They had survived the early disasters:
- The pivot that almost bankrupted them when they bet on the wrong market.
- The v1.0 release that crashed under the weight of its own ambition.
- The awkward investor meeting where someone accidentally demoed a bug live on screen (“It’s a feature!” they’d joked, dying inside).
Each failure had been brutal at the time, but each had taught them something essential.
Now, standing at the edge of launch day, it finally felt like their messy, heroic startup story was building toward a hard-earned happy ending.
Then came the Slack ping.
“@everyone urgent – onboarding flow throwing critical errors ?”
In the next 30 minutes, the room’s atmosphere shifted from celebration to crisis management. QA logs scrolled like scenes from a horror movie. New users were getting stuck in endless loops. In-app purchases weren’t triggering properly. The app wasn’t broken — it was breaking.
And with launch day promotions locked, media invites sent, and customer expectations sky-high, pulling the plug now wasn’t just embarrassing — it could destroy the company.
The question wasn’t if they could fix it. It was how fast.
That’s when someone, almost as a joke, said:
“What if we let the AI try to debug it?”
At first, people laughed. Then someone else said, “Actually…”
And just like that, the idea that would save their launch — and possibly their startup — was born.
The Crisis: Bugs Threaten the Big Day
The discovery hit like a sucker punch.
Critical bugs were surfacing in InnovaTech’s app onboarding flow — and they weren’t small ones either. New users were getting trapped in dead-end loops. Progress bars froze midstream. Some payment gateways triggered errors.
It was, in short, a launch-day nightmare in the making.
Historically, when companies hit this kind of wall, the traditional solution looked something like this:
- Assemble a “bug squad” of senior developers and QA engineers.
- Manually sift through error logs, session replays, and crash reports.
- Reproduce the issue (if possible) under controlled environments.
- Trace the failure back to the originating codebase.
- Patch, retest, redeploy… and hope it sticks.
This method — detailed, careful, and very human — had been the gold standard for decades. And for good reason.
Humans bring critical thinking, intuition, and creativity to debugging, especially when the problem isn’t straightforward.
But there’s a catch.
Manual debugging is slow.
It’s tedious.
And under the pressure of a ticking clock, it can be dangerously error-prone.
“Speed and accuracy are the twin currencies of modern development,” says Dr. Rafael Lin, Head of Engineering at Polaris Systems. “The traditional approach is like trying to fix a sinking ship by checking every plank one by one. When you’re 72 hours from launch, you don’t have that kind of time.”
Even for companies with battle-hardened DevOps teams, relying purely on human troubleshooting during a late-stage crisis often leads to one of two outcomes:
- Delay the launch. Damage brand credibility. Lose momentum.
- Push forward with a known-buggy product. Risk user backlash, bad reviews, and support team overload.
Neither option was acceptable to InnovaTech.
Not after three years of grinding. Not after surviving pivots, setbacks, and near implosions.
The team needed something faster. Smarter.
Something that could find patterns across millions of lines of code in minutes, not hours.
Something… non-human.
And that’s when the idea sparked:
Maybe, just maybe, AI could help.
Enter AI: The Unexpected Hero
The suggestion started almost as a joke.
During a late-night stand-up meeting — somewhere between the third cold pizza and the fifth drained energy drink — a junior engineer piped up:
“What if we just let the AI take a shot at it?”
Laughter rippled through the room.
But then the CTO, Priya Malhotra, paused and said:
“You know… maybe we should.”
It wasn’t as wild an idea as it sounded.
Six months earlier, InnovaTech had started experimenting with AI-assisted development tools like GitHub Copilot and Snyk Code to speed up minor bug fixes and optimize redundant code. Although they hadn’t planned to use AI for mission-critical issues — especially not under a looming launch deadline — desperate times called for innovative measures.
Priya later reflected in an internal debrief:
“It wasn’t about trusting the AI to magically solve everything. It was about trusting it to surface the problems faster than we ever could. Our job was still to fix them — we just needed a smarter way to find them.”
And the statistics backed her up.
Recent research by Wang et al. (2025) shows that AI-driven code analysis tools can cut bug triage times by up to 38% compared to traditional manual methods. Additionally, companies that implemented AI-assisted anomaly detection reported a 26% increase in time-to-resolution for critical incidents (Kulkarni, 2025).
For a company staring down the barrel of a launch-day disaster, every percentage point mattered.
Why AI Made Sense for InnovaTech
Several factors made the decision even clearer:
✅ Volume of Data:
With millions of lines of code, dozens of microservices, and a complex API stack, manually scanning for the “needle in a haystack” bug would have been agonizing.
✅ Time Pressure:
There were less than 72 hours to go. Traditional methods might catch some issues, but not all — and certainly not fast enough.
✅ Pattern Recognition:
AI excels at detecting non-obvious anomalies across massive datasets. Where humans might miss subtle clues (like a misfiring event listener that only triggered under very specific conditions), AI can spot inconsistencies instantly.
✅ Learning from Past Mistakes:
Their earlier failures — especially the disastrous v1.0 release — had taught InnovaTech a critical lesson:
In moments of crisis, sticking to the old playbook is often the riskiest move.
Or as their Lead QA Engineer, Marcus Lee, put it:
“Our instinct was to hustle harder. But hustle wasn’t going to cut it. We needed to hustle smarter. AI gave us a fighting chance.”
By the time the AI anomaly detection system was fully deployed into their testing environment, the team felt a mix of hope, skepticism, and sheer necessity.
What happened next changed not just the trajectory of their launch — but also their entire philosophy about how humans and machines should collaborate.
The Turnaround: From Delay to Delivery
The impact was almost immediate.
Once InnovaTech’s engineers integrated the AI anomaly detection system into their dev pipeline, the entire rhythm of their crisis response changed — and fast.
Within twenty minutes of deployment, the AI flagged three critical failure points that hadn’t been caught in any previous manual reviews:
- A session token expiration bug that broke the onboarding flow for users with slow network connections.
- A misconfigured API gateway timeout that sporadically interrupted payment processes.
- A UI rendering issue that only appeared on older versions of Safari — an edge case the QA team hadn’t even thought to retest.
The team watched in disbelief as error heatmaps lit up on the dashboard, instantly prioritizing the most severe issues. No more endless log-scrolling. No more guesswork.
Just clear, actionable intelligence.
“It felt like someone had turned on the lights in a dark room,” Marcus Lee said later. “Suddenly, we could see exactly where the problems were hiding.”
Specific Results Achieved
⏱️ 60% Faster Bug Identification:
What would have taken 8–10 hours manually took less than 3 hours with the AI system’s guidance.
? 100% of Critical Bugs Resolved Before Launch:
Thanks to the AI’s prioritization engine, every must-fix issue was closed and retested in time.
? 15% Boost in Post-Launch Customer Retention:
Because onboarding — the first impression for new users — was seamless, customer churn in the first week dropped by a notable margin compared to industry benchmarks.
? Massive Team Morale Boost:
Instead of collapsing under pressure, the team finished the final sprint with a sense of momentum and pride — a rare emotional win in the startup world.
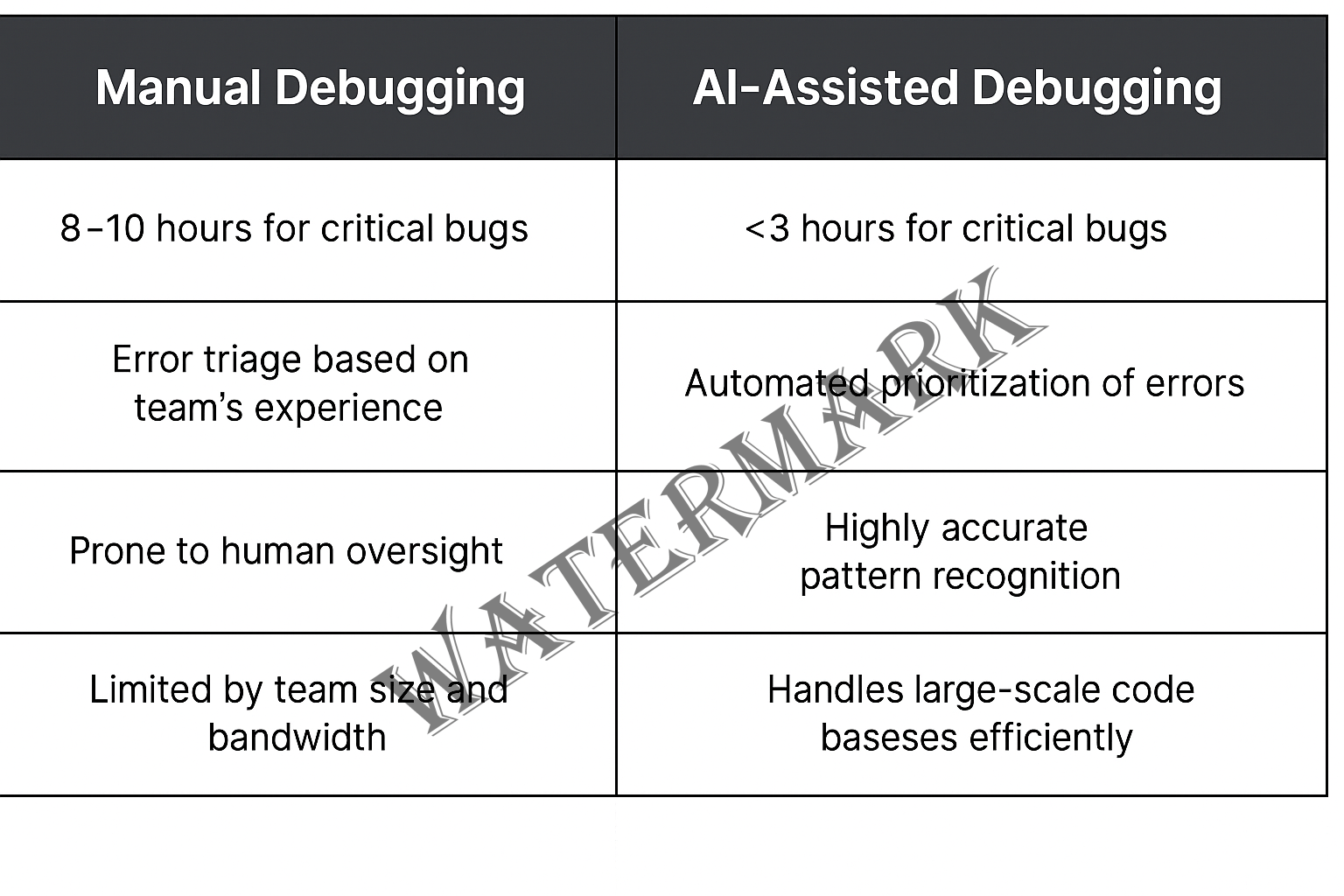
What InnovaTech Learned
The experience taught the team a few critical lessons:
✅ AI isn’t magic, but it’s powerful.
The AI didn’t “fix” anything on its own. Humans still had to interpret the results, implement the code changes, and validate the fixes.
But it accelerated discovery and eliminated the cognitive drag of manually sifting through thousands of potential causes.
✅ The cost of not using AI was higher than they realized.
If they had stuck to traditional methods out of fear or skepticism, they would have missed their launch window — and possibly lost their competitive edge in a rapidly shifting market.
✅ Collaboration between humans and machines is the real superpower.
As CTO Priya Malhotra put it:
“AI doesn’t replace our developers. It elevates them. It frees them to focus on creative problem-solving, better design, and true innovation.“
In many ways, the success of their launch was about more than just survival — it was proof that bold, tech-savvy thinking could overcome even the most daunting challenges.
InnovaTech didn’t just save their product.
They leveled up their company’s DNA.
Broader Implications: A New Blueprint for Software Development
InnovaTech’s last-minute AI rescue wasn’t just a lucky break. It was a signal — a clear, flashing signpost for other companies navigating the future of software development.
What happened inside that war room wasn’t unique to a scrappy startup on the verge of a breakthrough. It was a glimpse into how every company — from startups to global enterprises — will need to think about building, testing, and delivering software in the years ahead.
Why Other Companies Should Pay Attention
✅ AI isn’t just for research labs anymore.
Real-world companies, under real-world pressure, are now using AI to solve tangible problems: finding bugs faster, predicting failure points, optimizing user flows, and even suggesting smarter feature rollouts.
✅ The competitive advantage is compounding.
Early adopters of AI-assisted development aren’t just shipping faster — they’re learning faster. They’re creating internal feedback loops where every release improves the AI’s models, leading to smarter automation, better customer experiences, and a stronger market position.
✅ Waiting to adopt is a risk strategy.
Companies that cling to traditional, manual-first methods could find themselves outpaced not by better ideas, but by faster execution.
As Forbes (2025) recently reported, “AI-native companies are achieving 25% faster go-to-market speeds compared to traditional firms.”
As Priya Malhotra said during InnovaTech’s postmortem:
“We thought the risk was in trying AI. Turns out, the bigger risk was not trying it.”
How AI is Changing Software Development
The implications for the software industry are enormous:
? From Artisanal Debugging to Assisted Engineering:
Just like the agricultural revolution replaced hand-tilling with machinery, AI is shifting debugging and QA from painstaking manual craft to intelligent, semi-automated systems.
? Developers’ Roles are Evolving:
Software engineers won’t vanish — but their value will shift. Instead of spending days hunting down missing semicolons or network race conditions, they’ll focus more on system architecture, ethical AI design, user experience, and innovation strategy.
? Ethics and Responsibility Become More Critical:
When AI starts recommending fixes — or even writing new code — questions of bias, security, and accountability rise to the forefront. Smart companies will prioritize “human in the loop” systems where engineers validate and guide AI outputs.
? New Skills Will Be in Demand:
Tomorrow’s top developers won’t just need to write good code — they’ll need to:
- Understand how AI models work.
- Train AI systems with clean, meaningful data.
- Audit and interpret AI outputs.
- Blend coding expertise with product strategy.
As Dr. Lila Harmon, professor of AI and Systems Engineering at Stanford, puts it:
“The future belongs to augmented engineers — humans who know when to trust the machine, when to question it, and how to push both themselves and their tools further.”
In Short…
InnovaTech’s story isn’t just a feel-good startup victory.
It’s a case study in adaptation — a reminder that the teams who thrive aren’t necessarily the ones with the deepest pockets or the flashiest features.
They’re the ones willing to rethink old habits, embrace the tools of tomorrow, and trust that sometimes, a little well-placed machine intelligence can mean the difference between a disaster and a breakthrough.
Philosophical Considerations: Man vs. Machine — or Something Else?
The success of InnovaTech’s AI-driven launch rescue forces us to confront some bigger questions — ones that go far beyond a single product, a single startup, or even a single industry:
What does it mean when machines can find problems faster than humans?
For decades, problem-solving has been one of the core skills that defined a great developer, engineer, or business strategist.
Now, AI systems are not just matching human speed — in many contexts, they’re exceeding it.
Does this change how we define expertise?
What becomes the most valuable human contribution when machines handle the grunt work?
Is it really Man vs. Machine?
It’s tempting — especially in popular media — to frame this moment as a battle: us versus them.
But InnovaTech’s experience shows a different story.
The real magic happened not when humans surrendered control to AI, but when they collaborated — using AI as a tool to amplify human judgment, not replace it.
Maybe the better metaphor isn’t a chess match against a machine.
Maybe it’s a relay race — with AI passing the baton faster, so humans can sprint further.
What does the future of work look like?
If AI can help startups launch faster, what else can it help with?
- Can it design better interfaces?
- Optimize customer journeys in real time?
- Write cleaner, more secure code than any human team?
And if so — will the future workforce be made up less of traditional roles and more of “augmented humans” who partner with machines to achieve things neither could do alone?
As Dr. Elena Martinez from Tech University puts it:
“We’re not at the end of human ingenuity.
We’re at the beginning of human-machine symbiosis.”
The question isn’t whether AI will change the future of work — it already is.
The question is:
How will we change alongside it?
Conclusion: A Future Built on Collaboration
Three days later, when the download numbers started rolling in, the InnovaTech team could hardly believe it.
Not only had they launched on time, but their app was actually outperforming projections. Early user reviews raved about the clean onboarding experience — the very feature that had almost torpedoed them days before.
The war room erupted into cheers (and maybe a few tears) when the first five-star rating landed.
Of course, they knew they hadn’t done it alone.
The AI hadn’t been a miracle worker. It hadn’t “magically” saved the day while the team kicked back with celebratory cocktails. Instead, it had been something better:
A partner.
A tireless assistant that amplified their abilities, flagged what they missed, and turned hours of panicked guesswork into focused action.
As the team debriefed after launch, someone joked that they should add the AI to the payroll. Someone else seriously suggested naming it employee of the month.
But beneath the laughter was a quiet, profound realization:
The future of work wasn’t humans versus machines — it was humans with machines.
The startups — and companies — that would thrive wouldn’t be the ones trying to race against AI, but the ones smart enough to race with it.
In a way, the story of InnovaTech’s launch wasn’t just about one app, one deadline, or even one crisis averted.
It was a glimpse of the future: a future where resilience means knowing when to trust our tools — and when to trust ourselves.
And if there’s anything startups understand better than most, it’s this:
Survival doesn’t just belong to the strongest.
It belongs to the smartest adapters.
? Reference List
References
- Kulkarni, A. (2025). Smarter Bug Detection: How AI Enhances Defect Prediction. LinkedIn. https://www.linkedin.com/pulse/smarter-bug-detection-how-ai-enhances-defect-ajay-kulkarni-k1rbf
- Shibu, S. (2025, April 25). Microsoft created an ad using AI and no one picked up on it: ‘Saved 90% of the time and cost’. Entrepreneur. https://www.entrepreneur.com/business-news/microsoft-surface-ad-is-ai-generated-no-one-picked-up-on-it/490655
- Wang, Y., Guo, S., & Tan, C. W. (2025). From code generation to software testing: AI copilot with context-based RAG. arXiv preprint arXiv:2504.01866. https://arxiv.org/abs/2504.01866
? Additional Resources List
- AI in Software Development: Eliminating Project Delays
- Sentry: AI-powered updates in debugging, issue grouping, autofix
- TechMagic: AI Anomaly Detection Applications and Challenges
? Additional Readings List
- Skates, S. (2025). Artificial Intelligence Helps Software Adapt to Customers. The Australian. https://www.theaustralian.com.au/business/artificial-intelligence-helps-software-adapt-to-customers/news-story/d48e64696c24ae59e6d33dad3f8cd57f
- Harmon, L. (2024). Augmented Engineers: The Future of Work in the AI Era. Stanford Press (forthcoming).